As cyber threats become more advanced every day, it is now necessary to look to Artificial Intelligence Services (AI) and Machine Learning (ML) to protect systems and provide the best possible security for organizations.
AI is growing slowly and is starting to be used in many fields such as the medical industry or the car industry. It's time for AI to help fight against cyber attacks. With the advancement of technology and the lockdown, cyber security should be a top priority for businesses. Now more than ever, organizations from technology companies to social media websites are starting to use AI to stop cyber attacks.
By implementing AI in security systems, businesses can learn from the collected data and use it to their advantage. Along with AI, ML and security platforms, it can become a really powerful tool to prevent hackers and cybercriminals from stealing private data and information.
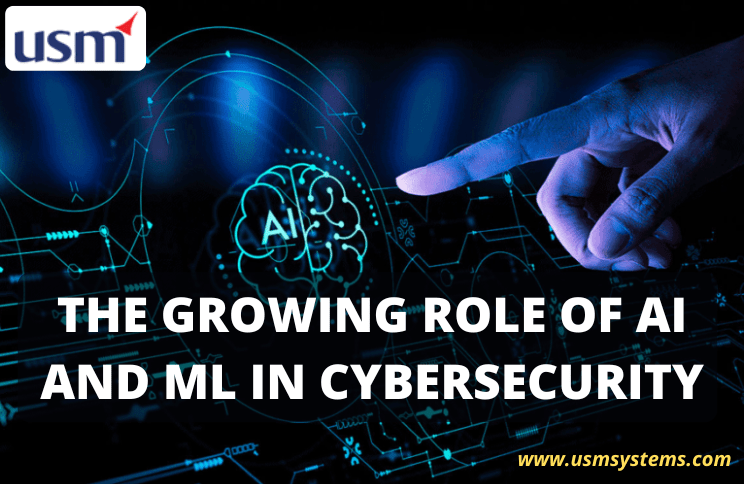
Machine Learning in Cyber Security
To enhance their security policies, cyber security analysts can apply ML in a variety of contexts. This makes it simpler for them to recognise, rank, handle, and eliminate fresh threats fast.
Automating tasks
Machine learning can be used in cyber security to accelerate repetitive tasks such as malware detection, vulnerability analysis, triaging intelligence and network log analysis.
Businesses can incorporate machine learning into their security workflow and accelerate response to incidents and mitigate them at a faster pace compared to manual human capabilities. Customers can automate repetitive tasks, which allows them to scale or downsize without increasing the number of employees, thereby reducing costs.
Classification and threat identification
Machine learning is used in applications to detect and respond to threats. This can be achieved by analyzing a large volume of security incidents to identify malicious behavior patterns. When such events are detected, ML uses a trained ML model to automatically deal with them.
You can create a database that feeds a machine-learning model using indicators of compromise (IOCs). These data sets help in real-time monitoring and threat detection, as well as response. Malware activity can be classified by using ML classification algorithms or IOC data sets.
Phishing
Traditional phishing detection algorithms cannot distinguish between malicious and innocent URLs. They are neither fast enough nor accurate enough.
Machine learning algorithms are used to predict URL categories and identify suspicious patterns. Models are trained using features such as email body data, punctuation marks, and more to distinguish malicious emails from malicious emails.
Webshell
WebShell is a block of malicious software installed on a website. It allows users to make modifications to the server's webroot folder. The attacker now has access to the database. A bad actor can access personal information.
Machine learning can detect normal shopping cart behavior and program the system to distinguish between malicious and normal behavior.
Network Risk Scoring
Quantitative methods that assign risk ratings to network segments help organizations prioritize resources. ML can be used to analyze previous cyber-attack data and identify which areas are most likely to be targeted by specific attacks.
This score can be used to estimate the likelihood and impact of an attack on a specific network area. This makes organizations less likely to be the next targets of an attack.
Human interaction
We are all aware of how well computers perform sophisticated task automation and issue resolution. AI is primarily concerned with computers, but it also needs people to provide orders and make informed decisions. We can infer that technology cannot take the role of people. Although machine learning algorithms are able to comprehend spoken language and identify faces, they still require people.
Read More: How AI is Transforming HR Management
What is the future of AI and ML in cybersecurity?
AI and ML will definitely play a major role in every field in the near future. In cybersecurity, this certainly allows organizations to better protect themselves, even as they look for sophisticated cyberattacks.
Humayun feels that an early detection system with capabilities to detect cyber-attacks early is a crucial part of cyber security. 'However', he says, 'just as AI and ML technologies are becoming more sophisticated, so are cyber-attacks'. One of the most complex challenges is finding a sufficiently flexible risk framework for assessing cyber risks. Indeed, it should be aligned with any law and insurance policies so that a sustainable solution is found.
Ivan adds that in the future, AI and ML will be used more widely. It becomes a tool to wade through massive amounts of data to detect malicious activity.
As more devices such as IoT come online, there will be wider vulnerabilities and more network traffic. As more and more businesses go through digital transformation, this gives 'greater opportunity for cybercrime and an even greater need for cybersecurity'.
Wrap it up
Although it is a useful tool, machine learning is not a panacea. As mentioned at the beginning of the article, as the machine learning space grows significantly, there will always be bad actors who develop their skills and technology to find and exploit weaknesses. Also, machine learning algorithms must remember to minimize their false positives, meaning they detect actions as malicious or part of a cyberattack.
Overall, technology is only as good (or bad) as the minds that use it. Therefore, it is imperative to combine the best technology and processes with industry experts to accurately and rapidly detect and respond to cyber threats. Would you like to integrate AI technology to avoid cyber-attacks and improve the overall security position of your business? Hire best artificial intelligence development companies in Newyork ?